Data-Centric NLP
🍂 Fall 2022 ⏰ Mon / Wed 10:00 am - 11:50 am 📍 WPH 203
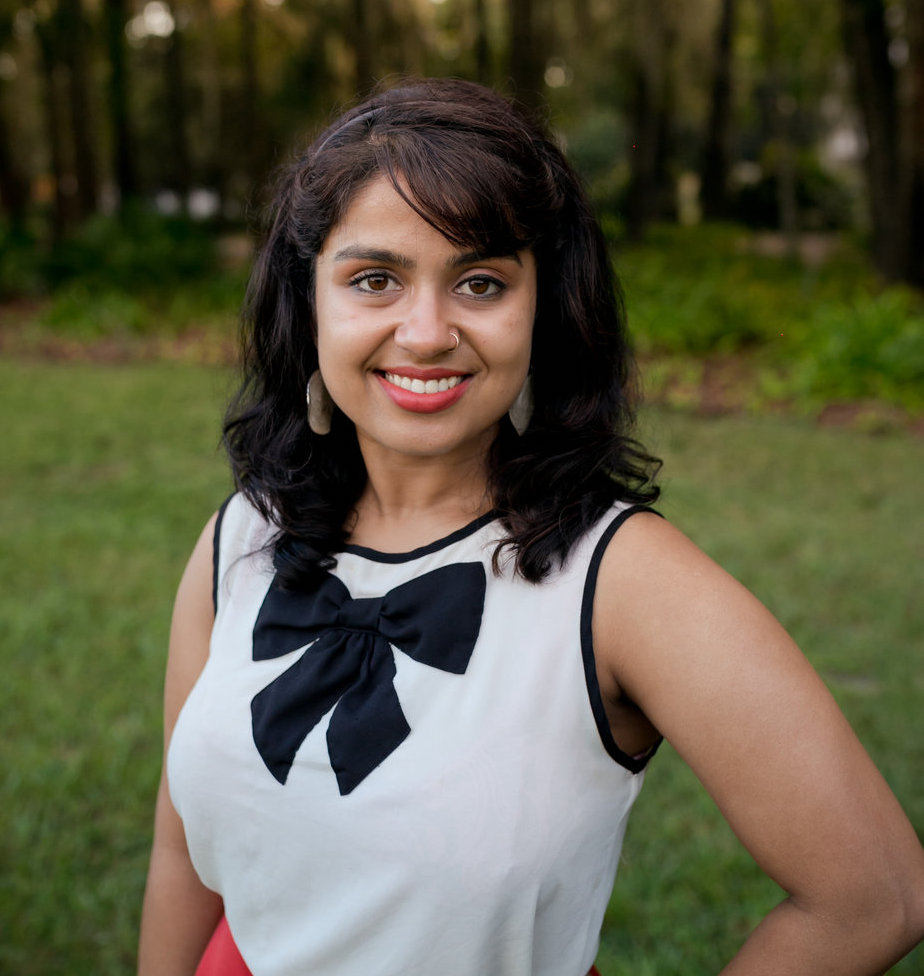
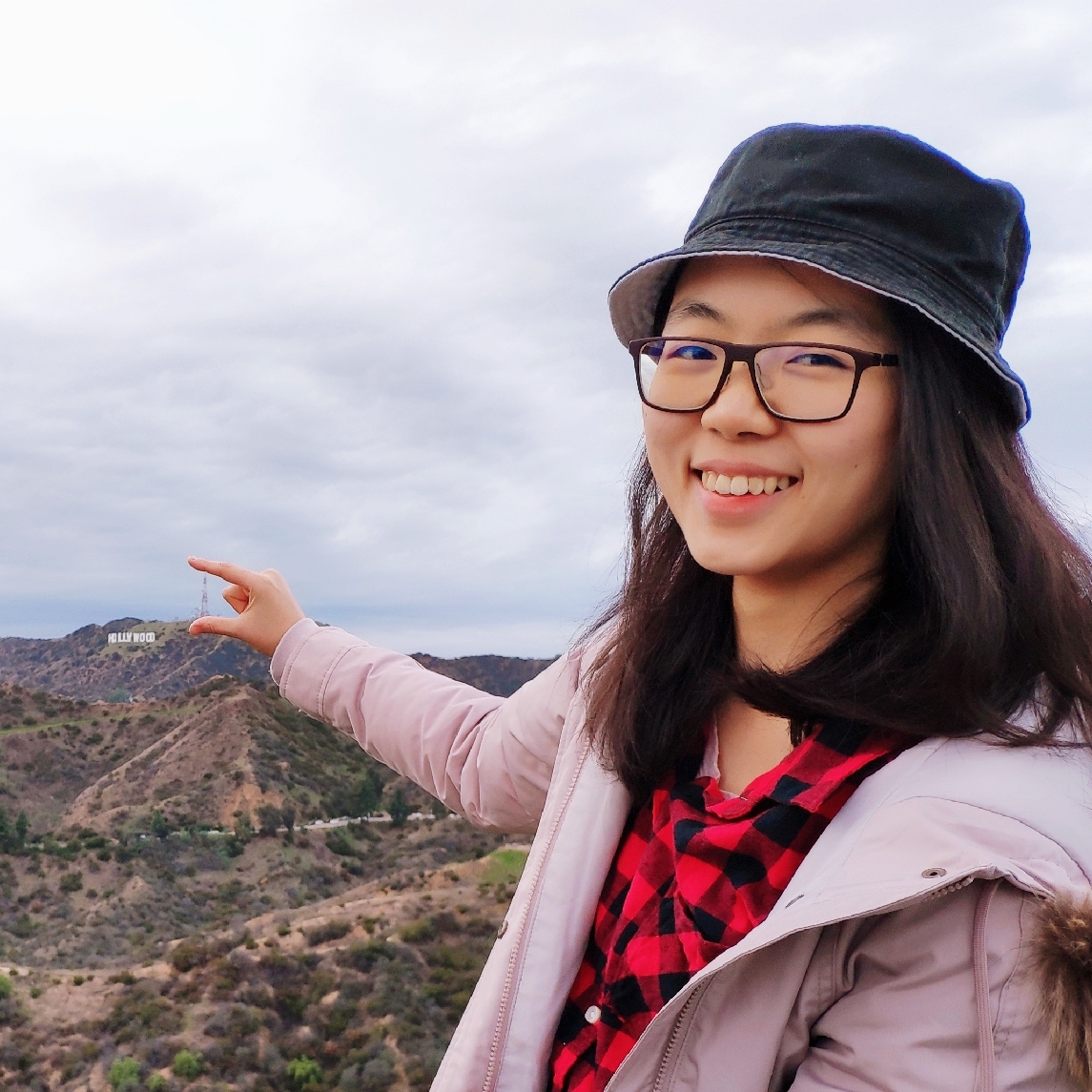
Teaching Assistant: Qinyuan Ye
Announcements
Nov 7: Week 12
- Feedback on your project progress reports has been shared, let me know ASAP if you did not receive yours.
- Project Presentations (see updated instructions) start on Nov 16.
- Intermediate scores will be shared by the end of this week.
- There will be no office hours on:
- 18th Nov (SoCal NLP)
- 25th Nov (Thanksgiving)
- 9th Nov onwards
Summary
While natural language processing (NLP) has been revolutionized by the advancement of deep learning, data still remains central in the development and application of NLP. Contrary to the common belief that data is just a necessary evil to be dealt with, this class will present datasets in NLP as central in designing tasks and models as well as measuring progress in the field of NLP. Starting with dataset creation methodologies and principles, we will survey the ethics behind dataset collection. This will lead us to discussing certain harms related to dataset collection, such as spurious and representational or social biases, along with data based techniques to address them. We will explore literature on how the quality of datasets can be estimated automatically, and how annotator disagreements and subjectivity are natural components of crowdsourcing. We will discuss questions on using data for accountability, where we will investigate how evaluation sets are built, as well as how model decisions are can be contextualized in data. We will discuss these concepts as they relate to unlabeled datasets. To wrap up, we will revisit data ethics towards better data citizenship.
Calendar
There are four class formats:
- lectures,
- 3 paper presentations (25 mins + 10 mins discussion, each),
- 2 paper presentations (30 mins + 10 mins discussion, each) + quiz, or
- final project presentation.
Datasets in NLP
- Aug 22
-
- Introduction and Overview
- Lecture
- Additional Readings
- Aug 24
-
- Data Collection and Data Ethics
- Lecture
- Additional Readings
- Aug 29
-
- More on Data Collection
- Readings
- Aug 31
-
- More on Data Ethics
- Readings
Biases and Mitigation
Estimates of Data Quality
- Sep 26
-
- Estimating Data Quality
- Lecture
- Additional Readings
- Sep 28
-
- Aggregate vs. Point-wise Estimates
- Readings
- Oct 3
-
- Anomalies, Outliers, OOD
- Readings
- Oct 5
-
- Disagreements, Subjectivity and Ambiguity I
- Readings
Oct 10-
- No Class
- Indigenous Peoples Day
- Oct 12
-
- Disagreements, Subjectivity and Ambiguity II
- Readings
Data for Accountability
Beyond Labeled Datasets in NLP
Privacy, More on Ethics, Outro
Outro and Project Presentations
- Nov 16
- Project Presentations
- Nov 21
- Project Presentations
Nov 23-
- No Class
- Thanksgiving
- Nov 28
- Project Presentations
- Nov 30
- Project Presentations
Dec 5-
- No Class
- Finals Week
Dec 7-
- No Class
- Project Final Report
Calendar is subject to change. More details, e.g. reading materials and additional resources will be added as the semester continues. All work is due on the specified date by 11:59 PM PT.
Assignments
There will be three components to course grades, see more details.
- Paper Discussion (20%).
- Class Participation (20%).
- Class Project (60%).
Students are allowed a maximum of 4 late days total for all assignments (not quiz sheets).
Note: Please read about the academic policies and a note about student well-being.
Pre-Requisites
Students are required to have taken undergraduate and/or graduate level classes in either Machine Learning or Natural Language Processing, equivalent to CSCI 544 (Applied Natural Language Processing) or CSCI 567 (Machine learning), or CSCI 662 (Advanced Natural Language Processing). Please email the instructor for special circumstances or specific clarifications.